Advanced Automotive Diagnostics Systems - From Diagnostics to Prognostics
Add bookmark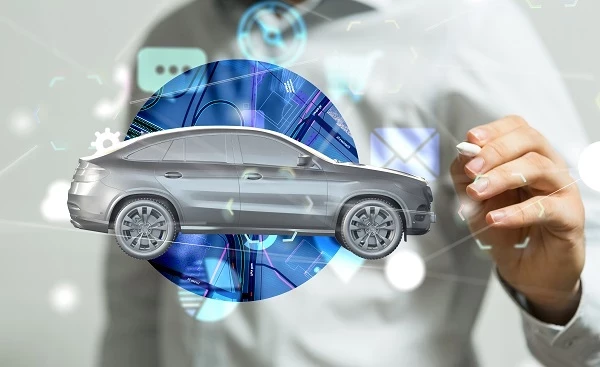
Connectivity and the Internet of Things are changing the landscape of the automotive industry, and as these technologies are gradually implemented, there are a number of interesting areas of innovation.
Remote diagnostics is one such area where technology is paving the way towards revolutionary new concepts of vehicle maintenance, including the use of artificial intelligence and deep learning neural networks to develop advanced prognostic systems.
Research by industry analysts Technavio in 2017 forecasts that the global automotive remote diagnostics market will grow at a CAGR of 16.79% during the period from 2017 to 2021. This is indicative of the progression towards prognostics - or predictive diagnostics - and away from traditional diagnostics.
The Drivers Behind Remote & Automotive Diagnostics
New vehicles, complete with 100 or more ECUs, have become highly complex networks and this increases the pressure to develop more efficient tools and systems to effectively diagnose the ECUs. The current trends in legislation and functionality offer opportunity but also come with risk as complexity increases. However, services such as ADAS and eCall are already taking advantage of connectivity and the push towards autonomous driving will see further development of car-to-X communication. The huge increase in the amount of data gathered from the vehicle and the ability to process it offers a variety of benefits in terms of maintenance.
One of these possibilities is vehicle diagnostics over the entire lifecycle, from engineering to manufacturing to after-sales. Technical experts could, for example, be based in a regional technical centre from which they could access data from an individual vehicle’s ECUs in order to diagnose faults. This information could be relayed to the service centre if local engineers are unable to diagnose locally.
This, however, is just the tip of the iceberg in terms of innovation. The continuous monitoring of of real-time data via a wireless network opens up a wide range of possibilities. Faults and problems could be determined in real-time and potential faults could be highlighted before they result in greater problems or damage. Drivers could be alerted to issues and directed to the nearest service centre, while information and diagnostics could be sent in advance to the local service centre so that they are prepared for the repair.
The cyclical data collected from a large number of vehicles also enables the monitoring of ageing processes across a large sample and can instruct preventative maintenance. Some manufacturers are already utilizing over-the-air software updates, and with more sophisticated data analysis and diagnostics, this is an area which offers huge potential savings in terms of recalls and warranty repairs. The introduction of remote diagnostics services also reduces the gap in the value chain and allows manufacturers to communicate directly with customers to offer better services.
Other driving forces behind remote diagnostic development include fleet management and MaaS (Mobility as a Service). For fleet managers, faster analysis of faults and issues leads to quicker action. This reduces downtime and means that vehicles can be repaired before problems escalate. Mobility as a service is gaining traction in large cities around the world as more consumers are moving away from ownership to shared services. Remote diagnostics will be crucial to passenger vehicle fleets which aim to provide a comprehensive and reliable service.
Cloud Diagnostics, Neural Networks and Artificial Intelligence
The next stage is cloud diagnostics, which combined with deep learning and AI, adds a new dimension to vehicle diagnostics. Software running in the cloud can manage campaigns for various fleets or models of vehicle. These campaigns can be targeted at specific vehicles in order to enable pinpoint vehicle programming and to highlight individual vehicle faults. For example, cyclical reading of the fault memory across a fleet means that troubleshooting and overall status can be checked automatically, while anomalies can be identified in real-time.
One of the biggest issues facing a number of industries is the problem of processing and analysing vast amounts of data in near real-time. With the ability to gather so much raw data from millions of vehicles, comes a need to filter and process it in order to undertake the appropriate actions. Engineers in a number of sectors are turning to artificial intelligence to solve this problem. Deep learning neural networks offer a solution to the problem of big data by ‘learning’ about the vehicle as data is analysed. Neural network techniques involve the ability to learn via the inputted data without the system being explicitly programmed. Data from vehicles can be streamed continuously to the cloud and AI algorithms will assess it in real-time. Algorithms can be trained to identify anomalies and detect patterns of behaviour based on the data sent from vehicle sensors. Artificial intelligence is already being implemented into autonomous test vehicles for the purpose of detecting and classifying objects, and it seems certain to play a role in the development of prognostics - particularly when autonomous cars hit the road.
Case Study: Softing Automotive Cloud
One prominent company in the diagnostics sector is Softing AG, which has developed its Automotive Cloud for the purposes of remote diagnostics, software-over-the-air (SOTA), measurement, testing, and communication. The system is designed to capture, prepare, and analyse data in the cloud for better diagnostics.
An acquisition system is located in the vehicle and is responsible for collecting the appropriate vehicle data. The system is configured in a way which makes it possible to store individual measurement values as local data, and is designed to run seamlessly on a vehicle’s embedded platform. A connected gateway (GW) or a powerful vehicle communication interface (VCI) is used to transfer collected data to the cloud for evaluation. The data transfer is based on widely approved communication standards with Transport Layer Security (TLS) encryption technology, mitigating the impact of instability from unreliable internet connections.
The transmitted data is stored in a database in the cloud, with aggregation and further analysis carried out by a cloud application. Various-vehicle specific profiles are used for identifying the correct type of data, allowing for simple interpretation. As well as receiving vehicle data, the cloud application also supports the execution of action, such as diagnostic tasks, triggered by inputs in the user interface and resulting in data transfer to the vehicle.
The cloud-based solution allows the intelligent collection and analysis of big data in the cloud. Data is processed and displayed graphically to show patterns and correlations and to present information in an easily digestible format. This information be accessed remotely via web services, while changes in vehicle condition can be communicated directly to the customer.
Summary
Advanced automotive diagnostics is an exciting area of auto-manufacturing because it offers a variety of benefits. The collection of data from a huge number of vehicles means that OEMs will have access to a wealth of information. This can be implemented to help reduce recalls and warranty repairs by highlighting specific issues. It also offers OEMs a direct line of communication with the customer.
In the future remote diagnostics benefitting from AI will be used to produce real-time models of vehicles in order to develop preventative maintenance routines. This will be a useful tool for fleet managers and developers of MaaS enterprises, as they look to reduce downtime and repair costs and streamline their services. It will also be critical as autonomous vehicles begin to penetrate the market. For driverless vehicles to be on public roads, they must have integrated safety systems which ensure perfect operation.
Cloud-based systems such as Softing’s automotive cloud will play a key role in the development of remote diagnostic systems, as ever-more data is transmitted and analysed in real-time in the cloud.
Sources:
https://www.futuremarketinsights.com/reports/automotive-remote-diagnostic-market
https://www.technavio.com/blog/benefits-remote-diagnostics-matters-automotive-industry